Research summary of: Loftus, T. J., Shickel, B., Balch, J. A., Tighe, P. J., Abbott, K. L., Fazzone, B., Anderson, E. M., Rozowsky, J., Ozrazgat-Baslanti, T., Ren, Y., Berceli, S. A., Hogan, W. R., Efron, P. A., Moorman, J. R., Rashidi, P., Upchurch, G. R., & Bihorac, A. (2022). Phenotype clustering in health care: A narrative review for clinicians. Frontiers in Artificial Intelligence, 5.
Key Takeaways
- Human pathophysiology is highly complex and often requires flexible analytic methods to process and clarify that complexity.
- Clustering algorithms represent a valuable approach for identifying subgroups of patients or conditions that can advance our understanding of disease mechanisms and treatment responses and optimize future research efforts.
"Applied properly, patient and disease phenotype clustering can reveal obscured associations that can help clinicians understand disease pathophysiology, predict treatment response, and identify patients for clinical trial enrollment."
Summary
The questions covered:
- How do clustering algorithms work?
- What types of clustering algorithms exist, and what considerations go into selecting the optimal clustering algorithm for a particular healthcare need or research question?
- What are the most promising opportunities for clustering to enhance healthcare delivery and research?
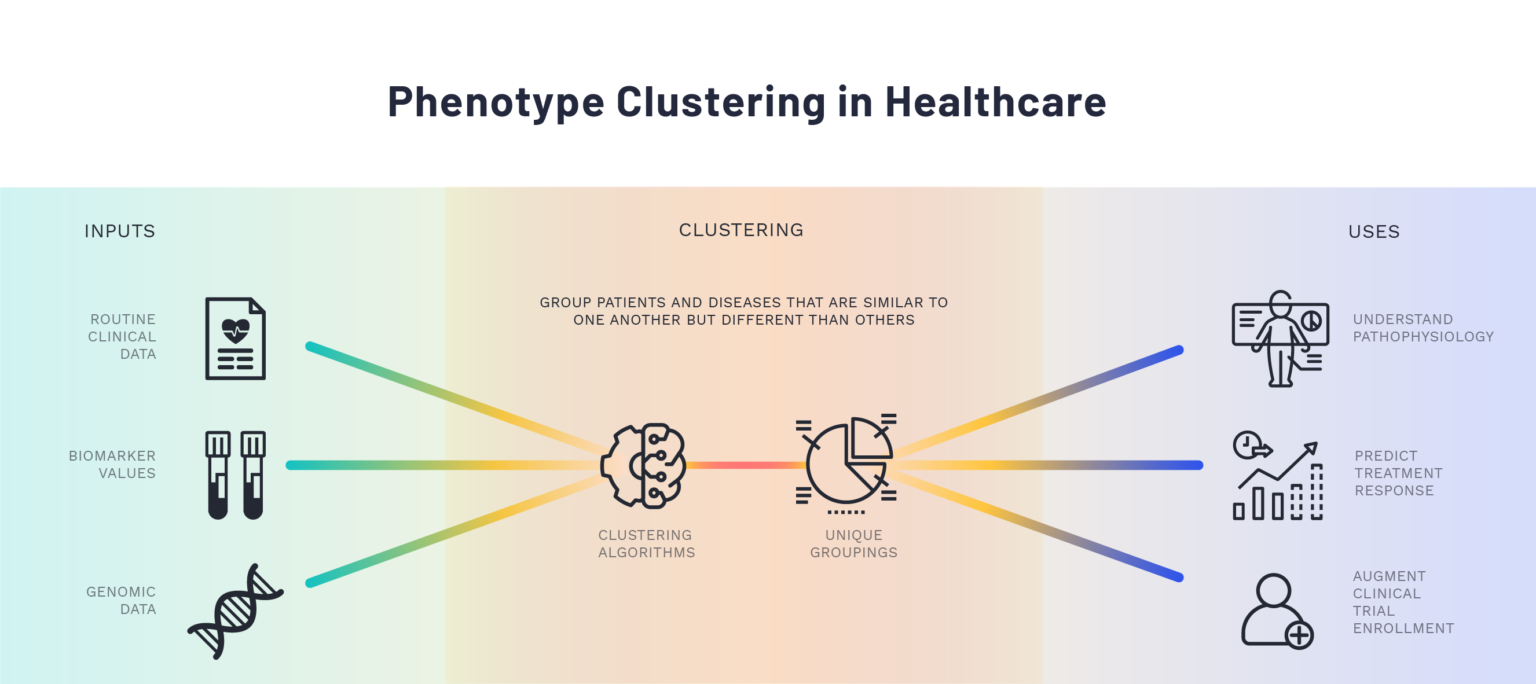
Clustering algorithms are data-driven methods for assessing which objects are most similar to one another based on one or more elements. They can be used to identify meaningful subgroups of patients or diseases through the input of clinical or physiological data without requiring a prior hypothesis about how the data points or variables relate to each other. Additionally, while traditional statistical methods rely on the assumption that associations between variables are linear and additive in nature, clustering algorithms allow for the possibility of non-linear relationships and higher-order interaction effects. Though many different clustering algorithms are available, they follow a uniform conceptual approach and differ primarily in how they define similarity for the patients or objects of interest.
The data-driven, flexible nature of cluster analysis offers opportunities for insight and improvement in many areas of healthcare. The authors highlight several examples from the published literature of advancements in our understanding of complex diseases, such as acute respiratory distress syndrome and sepsis, driven by cluster analyses. The potential applications for this methodological approach in movement health are extensive and expanding, given the advancements in technology affording increasing granularity and precision in measurement capability. Indeed, the innovative nature of many of the newly-available movement health measures requires such flexible, hypothesis-generating analytic methods.
The article provides general instructions for common data preprocessing steps to optimize cluster analysis, as well as an overview of the relative strengths and weaknesses of particular clustering algorithms to guide the selection of the appropriate approach for a specific dataset or research question. At the same time, however, the authors caution that the effective use of these methods relies on a combination of statistical or methodological expertise and domain knowledge of the underlying biological system. When utilized effectively, clustering algorithms provide unique insight into complex clinical challenges and offer solutions for improved pathophysiological understanding and healthcare delivery.
Abstract
Human pathophysiology is occasionally too complex for unaided hypothetical-deductive reasoning and the isolated application of additive or linear statistical methods. Clustering algorithms use input data patterns and distributions to form groups of similar patients or diseases that share distinct properties. Although clinicians frequently perform tasks that may be enhanced by clustering, few receive formal training and clinician-centered literature in clustering is sparse. To add value to clinical care and research, optimal clustering practices require a thorough understanding of how to process and optimize data, select features, weigh strengths and weaknesses of different clustering methods, select the optimal clustering method, and apply clustering methods to solve problems. These concepts and our suggestions for implementing them are described in this narrative review of published literature. All clustering methods share the weakness of finding potential clusters even when natural clusters do not exist, underscoring the importance of applying data-driven techniques as well as clinical and statistical expertise to clustering analyses. When applied properly, patient and disease phenotype clustering can reveal obscured associations that can help clinicians understand disease pathophysiology, predict treatment response, and identify patients for clinical trial enrollment.